The return from pharmaceutical innovation has seen a steady decline over the past few decades. Sales per asset continues to grow at a slower pace compared to the cost of discovery, development and manufacturing of drugs. Artificial intelligence (AI) based technologies have emerged as a beacon of hope for the pharma industry. Use of AI in pharma applications has the ability to provide data-driven solutions to persisting problems.
The use of AI is helping drive greater efficiencies in the pharma industry by providing an additional boost to creativity with better technology-aided processes. It also leads to reduced timelines and enhanced data processing, while the accuracy of machine learning (ML) minimizes losses due to human error. By reducing the overall need for manual intervention, AI-based solutions are helping reduce costs of R&D in pharma through large scale and more focused research.
Pharma-focused AI startups are not only attracting attention from big pharma, but also VC firms and technology conglomerates. In January 2020, investment into AI in pharma reached $5.2 billion. Read on to find out the key companies that have placed their bets on use of AI in pharma.
Big pharma’s AI initiatives
Big pharma companies have made great strides in adopting AI in pharma applications. Some of them, including Johnson & Johnson, AstraZeneca, Bayer, Merck, Novartis and GSK, are part of a life sciences consortium in Europe called Mellody (Machine Learning Ledger Orchestration for Drug Discovery), which is the first initiative that connects data from the world’s largest pharma companies to support AI-powered drug discovery.
- Johnson & Johnson’s innovation laboratories, JLABS, is studying new technologies in a program series called Quickfire Challenges, with the goal to help companies overcome the challenges in the adoption and application of AI and other emerging technologies.
- AstraZeneca has announced that it’s creating an AI innovation center in China to use AI-based digital technology in R&D, manufacturing, operations and commercialization to accelerate the delivery of medicines to patients globally. Similarly, GSK is creating a global data-focused AI and ML team for drug R&D.
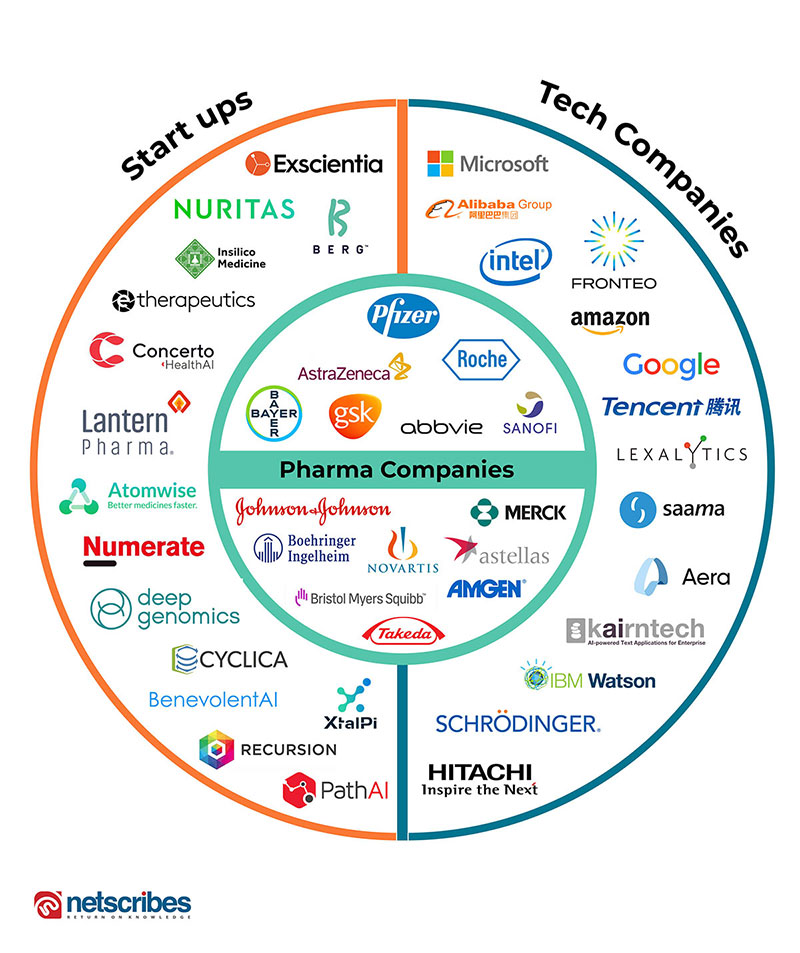
Leading companies for AI in pharma
To stay ahead in the race to drive innovative AI-driven solutions, many pharma and biopharma companies are partnering directly with AI startups or with tech companies that specialize in the technology. For instance, AI-based firm Exscientia has collaborated with companies like Bayer, GSK and Sanofi. Meanwhile, Microsoft has partnered with companies such as AstraZeneca and Novartis for leveraging AI in pharma R&D.
Major AI applications
With the volume of medical data growing at an astounding rate, AI is helping the pharma industry utilize it to improve efficiencies and optimize the overall cost of drug development. Furthermore, the demand for huge data processing and efficient service delivery has put AI-enabled solutions at the forefront of the pharma revolution. While its initial applications may have been concentrated in R&D, AI is now rapidly penetrating various segments of the pharma industry.
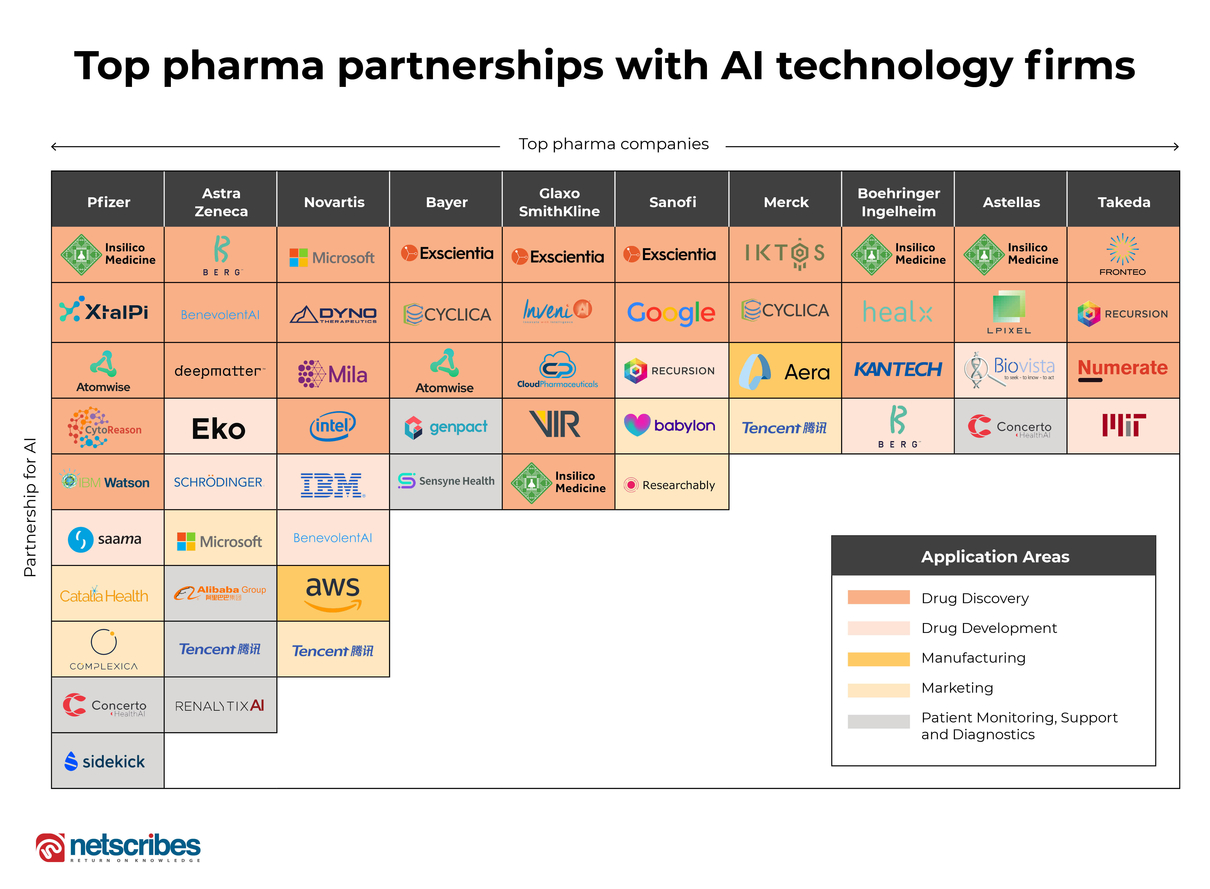
AstraZeneca has notably been the most welcoming towards leveraging AI, including it in every step across the chain of R&D and post-commercialization processes. Pfizer and Novartis come a close second, with the former having yet to tap into AI in pharma applications in drug manufacturing, and the latter, in patient monitoring and support.
Now, let’s dive deep into some of the key applications of AI in pharma:
1. Drug discovery
This phase of R&D is focused on finding new drug molecules effective against a particular diseased biological target. According to a study by the Tufts Centre for the Study of Drug Development, the estimated discovery and development cost of each FDA approved drug is around USD 2.6B. The discovery process usually takes the longest, as scientists have to run thousands of experiments before settling in on the right disease target.
The predictive models made for finding new molecules and compounds are prone to inaccuracies due to human error. This consists of incorrect data processing or miscalculations. The entire process, with its slow progress and an increasing number of failures, ultimately lead to the sky-high costs.
The use of AI in drug discovery can expedite the overall process. Applied intelligence can improve drug discovery success rates by 8-10%, resulting in savings worth billions of dollars for the industry. Finding drug discovery compounds and precision medicine are the major trending applications over other pharma-based AI applications.
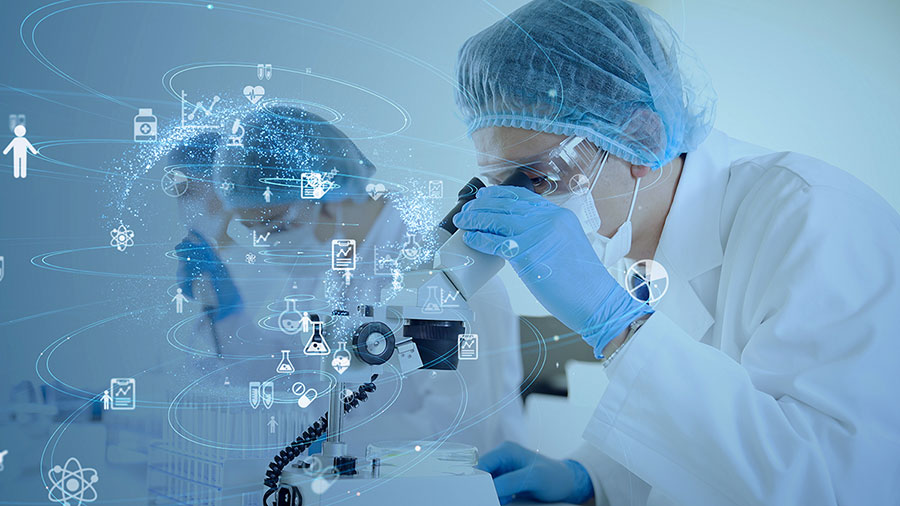
In fact, pilot projects on AI-based drug discovery tools have demonstrated a significant reduction in development time. Andrew Hopkins, the CEO of Exscientia, an AI-based drug discovery company, has claimed that their specialized process can reduce the time spent in drug discovery from 4.5 years to as little as 12 months, and reduces discovery costs by 80%.
Boehringer Ingelheim has partnered with Insilico Medicine to use AI technology in identifying potential therapeutic targets. Janssen Research & Development has also collaborated with software solutions provider nference to apply AI in the identification and selection of new targets and disease subsets to aid therapeutic programmes.
AI can be used to find candidate molecules for drugs, develop compounds from scratch, and aid the process of synthesizing the molecules, with better efficacy. Merck KGaA and AI start-up Iktos, are using generative modelling AI for rapid and cost-effective discovery and design of new compounds. Meanwhile, Takeda has partnered with drug-discovery firm Recursion for AI-powered examination and identification of novel preclinical candidates for rare diseases.
AI also helps in better understanding the disease’s mechanisms. For instance, Astellas Pharma has leveraged an AI-instilled image analysis solution known as IMAC Lab, from LPIXEL, a leader in image analysis and life-sciences technology. They are working on cell selection and management processes involved in researching regenerative medicine and cell therapy.
2. Drug development
Drug development through clinical trials runs a high risk of failure due to human errors in data processing and candidate monitoring. The timeline of clinical trials also runs longer, which ultimately delays its commercialization. Clinical trial data can also be misinterpreted sometimes due to human error from lack of knowledge or attention, which adds to the factors leading to its failure.
AI systems and algorithms process vast amounts of information quicker and with precision, maintain proper records and ensure transparency when it comes to clinical trial data. Through its data-driven decision making, AI not only reduces the entire timeline of drug development, but its accuracy also ultimately improves drug approval rates, and minimizes loss. It can be used to optimize the entire trial process, including trial designing and site selection.
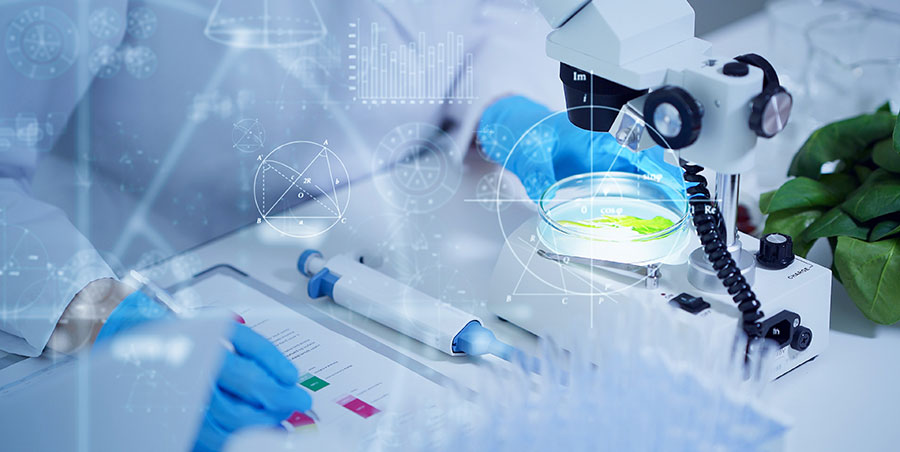
For instance, IBM Watson’s Clinical Trial Matching (CTM) eliminates the need to manually compare clinical trial enrollment criteria. CTM uses AI to read through patient medical data and match the right patient to the right clinical study. This has reduced the pre-screening wait time by 78% during the trial period (around 16 weeks) and automatically eliminated 94% of patients who failed to meet clinical trial requirements.
AI also enables faster and accurate gathering of clinical data, identification and monitoring suitable candidates for trials, predicting risk and toxicity, and monitoring drug adherence in trial candidates. Novartis has leveraged BenevolentAI’s technology and data science platform to manage clinical trial data and monitor drug adherence in candidates.
3. Manufacturing
The drug manufacturing process, again, can take longer when not optimized through technology. The use of manual processes and spreadsheets in the supply chain is still prevalent in the pharma industry, which require human intervention. It ultimately leaves room for error due to inaccurate data entering and processing, guesswork, and failed outcomes. Such errors can lead to manufacturing failures and huge losses.
AI can be used for pharma quality control, reducing design time, inventory management, predictive maintenance, demand forecasting, logistics optimization and end-to-end-visibility. Amgen has developed an AI-powered process that has greatly enhanced its ability to find patterns in manufacturing deviations and to prevent their recurrence.
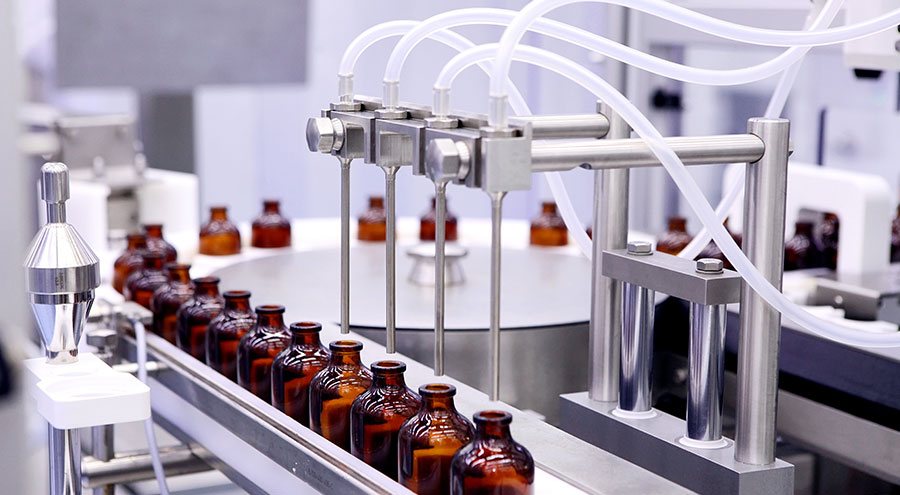
AI also makes the entire manufacturing process more accurate through proper planning of the supply chain. Merck KGaA has leveraged AI startup Aera Technology’s platform to help support its decision-making, through predictive and prescriptive supply chain modelling. Meanwhile, Novartis has partnered with Amazon Web Services (AWS) to digitally transform the manufacturing, supply chain and delivery of its medicines using cloud services.
4. Sales and marketing
End-to-end visibility provided by AI for drug commercialization is a great value-add for pharma companies. AI can help pharma companies coordinate product launches better, provide physician decision support and marketing operations, predict market access, and aid pricing decisions.
Pfizer Australia is developing AI-based digital analyst tools from technical consultancy firm Complexica to improve their sales and marketing decision making.
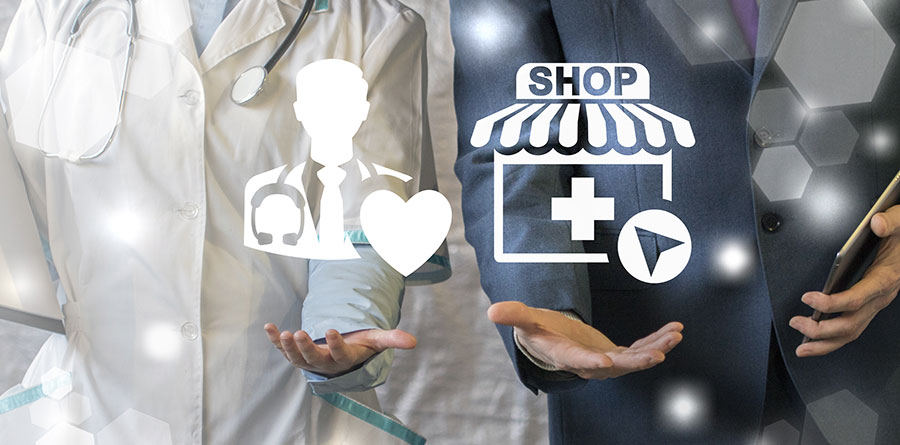
Patient engagement powered by AI is a leading field in pharma marketing, which not only provides guidance to patients, but also ensures a positive experience.
- Sanofi has partnered with video consultation business Babylon, to offer an online AI Health Service for those who suffer from digestive health issues.
- Novartis has co-developed an AI Nurse with Tencent Holdings, as a needs-led platform supporting patients, physicians and nurses to better manage heart disease.
Some pharma marketers are using AI to understand customer journeys at a deeper level. Johnson & Johnson uses an AI-powered virtual-assistant, Andy, to guide its US customers throughout their ACUVUE brand contact lens journey, mapping the entire process starting from new users to long-term ones. This enables them to deliver more personalized experiences across the buying journey.
5. Remote patient monitoring and support
After the launch of their drugs, pharma companies may also need exchangeable health data to track and engage with patients remotely. Patient monitoring helps them develop more precise drugs and therapies.
Applying AI towards patient safety is extremely important, particularly in detecting potential adverse effects of their drugs in real-time. Pfizer, with robotics company Catalia Health, has announced the upcoming launch of a one-year home robot program that uses voice interactions powered by conversational AI to assess a user’s mood, record data, manage symptoms, and provide helpful information about prescription drugs. Pfizer has also teamed up with SidekickHealth to launch a digital medication management platform across Europe.
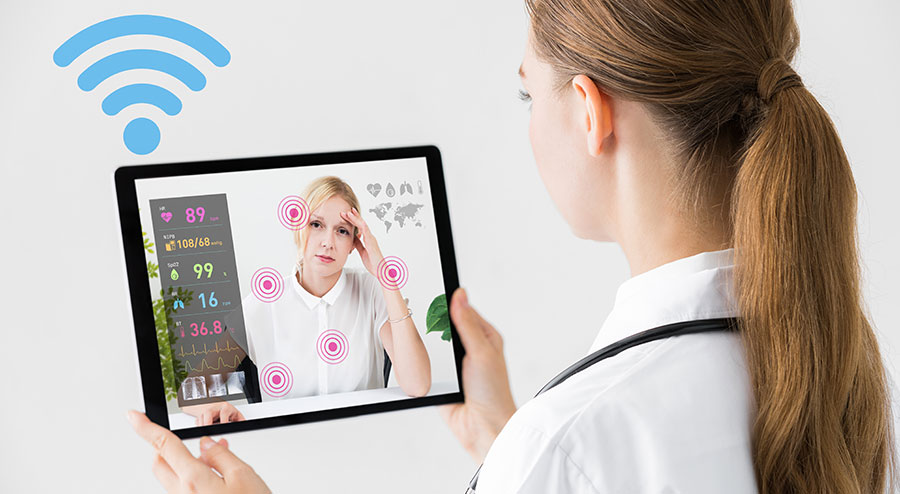
AI-enabled patient support programmes and diagnostics can transform pharma’s relationship with its customers, improve drug adherence and retention, aid disease prevention and deliver better patient outcomes. MERCK KGaA has collaborated with Johnson & Johnson to develop an AI tool for detecting diseases affecting low-income regions of Africa, Asia, and North and South America. Such developments could potentially decrease the global disease mortality rate.
Looking ahead
AI is guiding pharma companies towards a new era of innovation. This technology is slowly acquiring more success stories across the industry. For instance, GSK and Exscientia, through their collaboration, have developed the first-ever AI-powered drug candidate, to treat patients with Chronic Obstructive Pulmonary Disorder (COPD). The drug, which has moved forward into human trials, shows promise.
AI technologies, thus, have great potential to address declining ROI in the pharma industry. AI firms are continuously working on advancing their technologies, while addressing issues such as cybersecurity, transparency and data regulations, which can hinder its adoption. Despite the potential it contains, not all pharma companies have the resources or skill-set to access these technologies. With advancement in AI, a potential solution which can simplify its complex nature, and perhaps reduce its cost, to further improve ROI, is the need of the future.
Netscribes’ healthcare market intelligence solutions enable companies in the pharma industry to understand the current market landscape and stay prepared for developments on the horizon. Contact us to know more.